Detecting invisible roads
- Space4Good
- Jul 25, 2024
- 3 min read
Using a super-resolution algorithm on medium-resolution satellite imagery
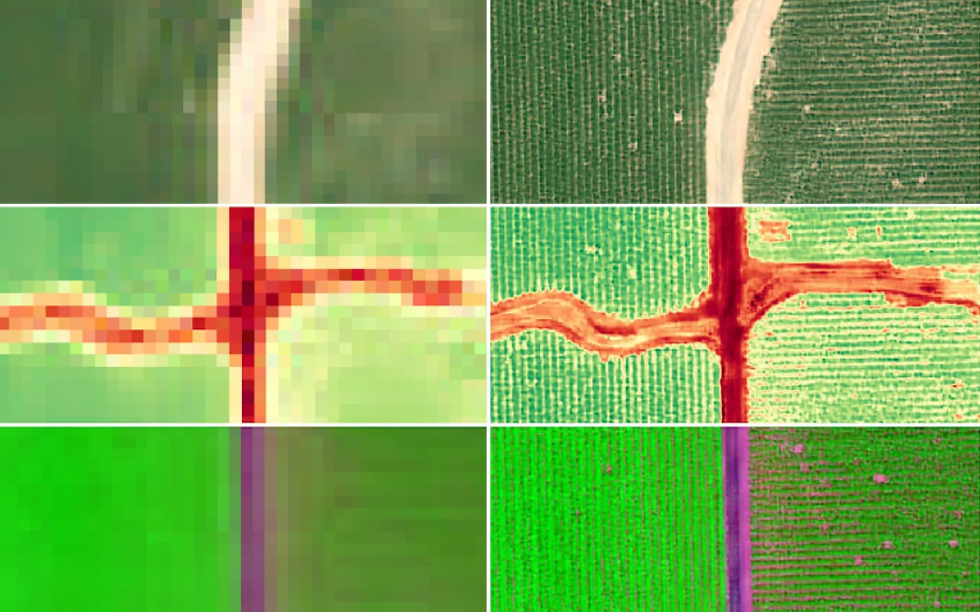
Figure 1. Sentinel-2 Deep Resolution 3.0. Source: Akhtman, Y. (2024, May 2). Medium.
Space4Good and Wageningen University and Research (WUR) have a history of collaboration by providing student opportunities within the business context, via internships and research opportunities. The most recent collaboration occurred within the context of an Academic Consultancy Training (ACT). Within the course Remote Sensing and GIS Integration, students not only gain academic knowledge but also learn about the challenging world of geo-business. The main part of the course involves working with a team on a consultancy project for an external client.
Space4Good contributed with an opportunity for students to work in a road detection project. A team of six master's students enrolled in the Geo-Information Science program were up for the challenge: Jack Bakker, Gabrielė Tijūnaitytė, Dong Liang, Tristan van Stee, Qin Xu, and Gert-Jan Groot Wassink.
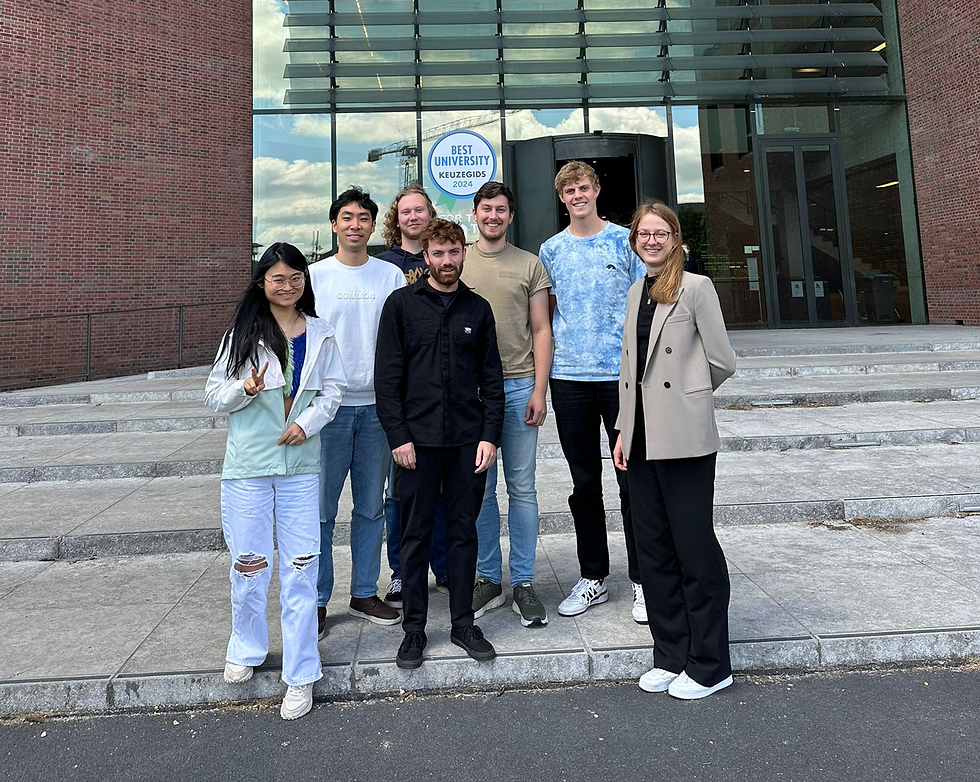
Figure 2. ACT student team and S4G project supervisor Federico Franciamore at Wageningen Universiteit.
Remote Sensing Opportunities for Forest Resources
The illegal exploitation of forest resources poses a global threat to ecosystem services, biodiversity, and the livelihoods of millions of people. The presence of roads can be a clear sign of potential illegal activities in an area, as they are often constructed for logging, mining access, and poaching. Therefore, it is crucial to detect the emergence of small roads in order to monitor and combat such illegal activities.
Remote sensing technologies offer the potential to detect roads in forests; however, the detection of small-scale features such as roads using open-source satellite-based remote sensing data is limited by insufficient spatial resolution. The focus is on exploring the feasibility of enhancing the spatial resolution of available open-source data, particularly Sentinel-2, and developing techniques for road detection using this enhanced data.
ACT Project Goal
In our project, we aimed to explore the potential for detecting smaller-scale roads in medium-resolution satellite imagery, specifically Sentinel-2. We utilized the readily available Deep Learning algorithm known as S2DR3.0 model (Sentinel 2 Super Resolution 3.0) to enhance the resolution of Sentinel-2 imagery from 10-60m to 1m. Our primary focus was to assess whether this approach could enhance the accuracy of road detection models.
Therefore, the main goal of our project was to determine if a deep-learning-based super-resolution model could improve road detection performance in tropical rainforests using Sentinel-2 imagery, focusing on two study areas in Indonesia: Kiani and Itci. This primary objective comprised four sub-objectives, all of which we have completed:
1. Applying a super-resolution algorithm to cloud-free S2 imagery to obtain upscaled Sentinel-2 (S2+) imagery.
2. Creating and preparing a road annotation dataset for the study areas.
3. Developing two deep learning models for road detection trained on S2 and S2+ imagery, respectively.
4. Assessing the quality of both road detection models and comparing them.
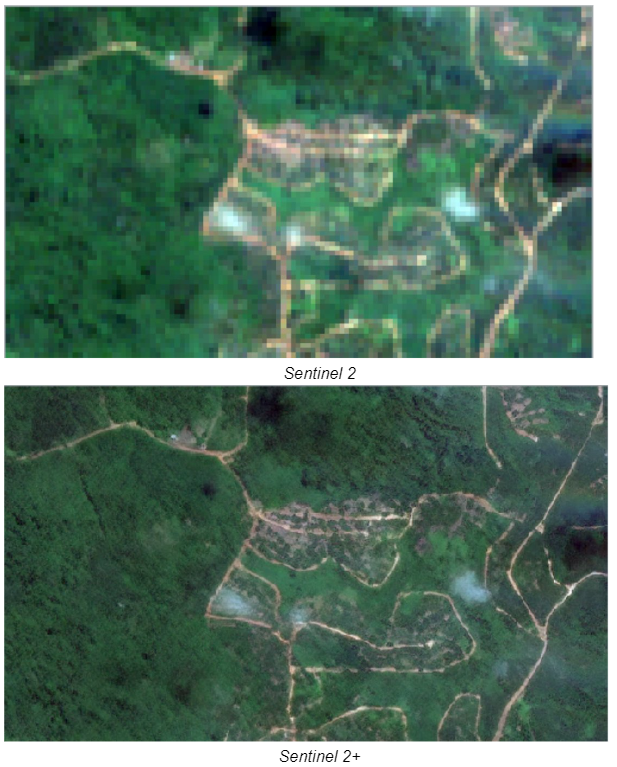
Figure 3. Visual Inspection of Sentinel 2 imagery (S2-top) and Sentinel-2 super-resolution (S+2-bottom)
Road Detection Models
To determine whether artificially upscaling imagery is useful, we built two road detection models based on Deep Learning pixel-wise classification: one for original Sentinel-2 imagery (S2) and another for artificially upscaled imagery (S2+).
Comparing model performance was a challenge due to the different spatial resolutions of the road presence predictions. We addressed this by developing a strategy to match resolutions (see Figure 2), and then compared the predictions to the ground truth (GT) road rasters to gain insights into the differences in model performances.
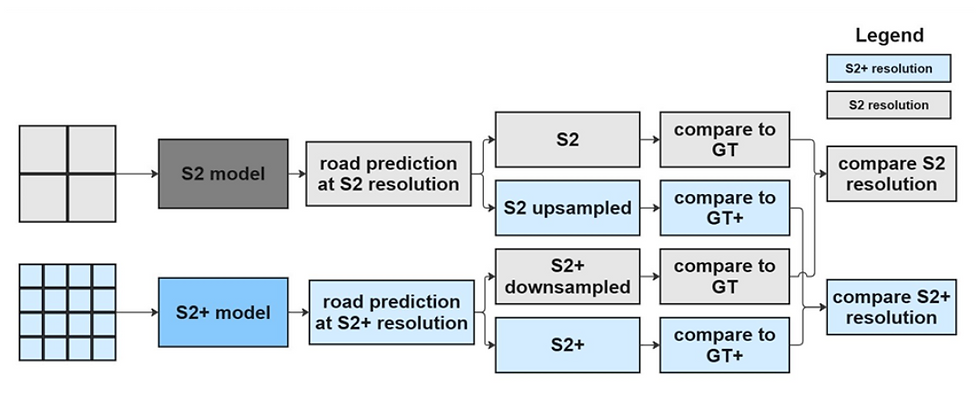
GT - coarse ground truth data (road raster); GT+ - fine ground truth road raster;
S2 - Sentinel-2; S2+ -Sentinel-2 super-resolution.
Findings
Results images below showed that using artificially upscaled images which is S2+, the road detection model performed much better at predicting road pixels than the original sentinel 2 images.


Figure 5. Road detection and prediction of Sentinel 2 imagery (S2-top) and Sentinel-2 super-resolution (S+2-bottom)
More information can be found in this storymap.
Next Steps
Although the project only lasted 15 work days, there is still ample room for improvement should more time be available. Despite proving that the S2DR3.0 model is beneficial for road detection in forests, there are many super-resolution models available. Trying out different models or creating a new company-owned model by Space4Good is also a viable option.
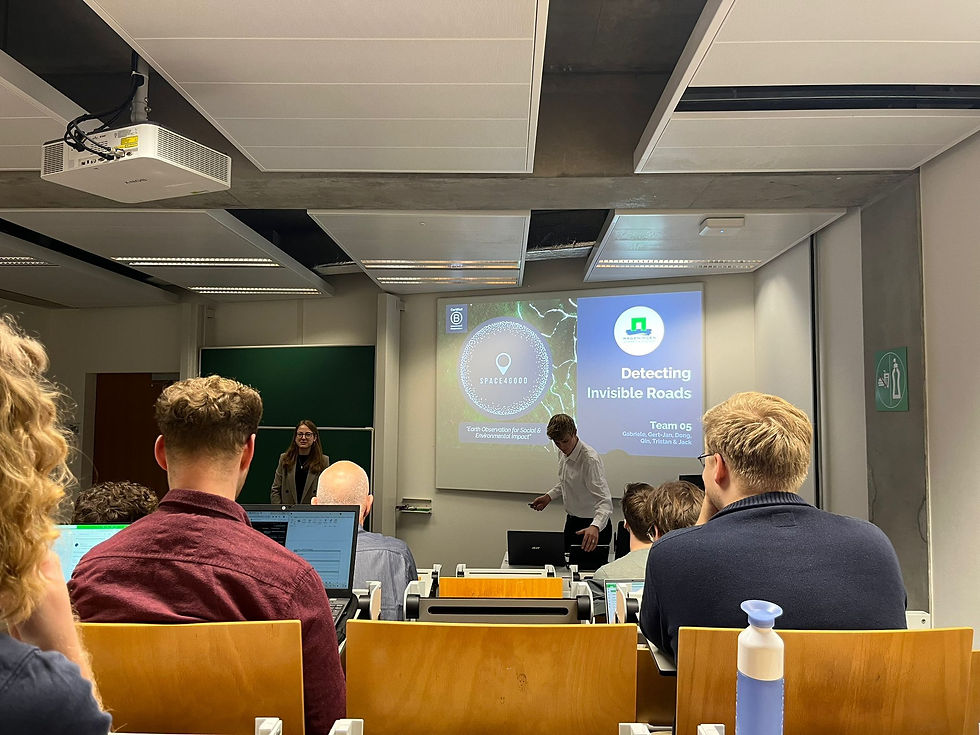
Figure 6. Final presentation "Detecting Invisible Roads" at Wageningen University
Thank you to the ACT students for providing an innovative piece for this month’s blog!
---------
This blog was written by the ACT student team comprised of Jack Bakker, Gabrielė Tijūnaitytė, Dong Liang, Tristan van Stee, Qin Xu, and Gert-Jan Groot Wassink, with edits from the Space4Good team.
Would you like more information or are you interested in collaborating with Space4Good? Visit our website or contact us via hello@space4good.com.
コメント